AI Customer Behavior Prediction in 2025: How to Stay Ahead of Your Customers
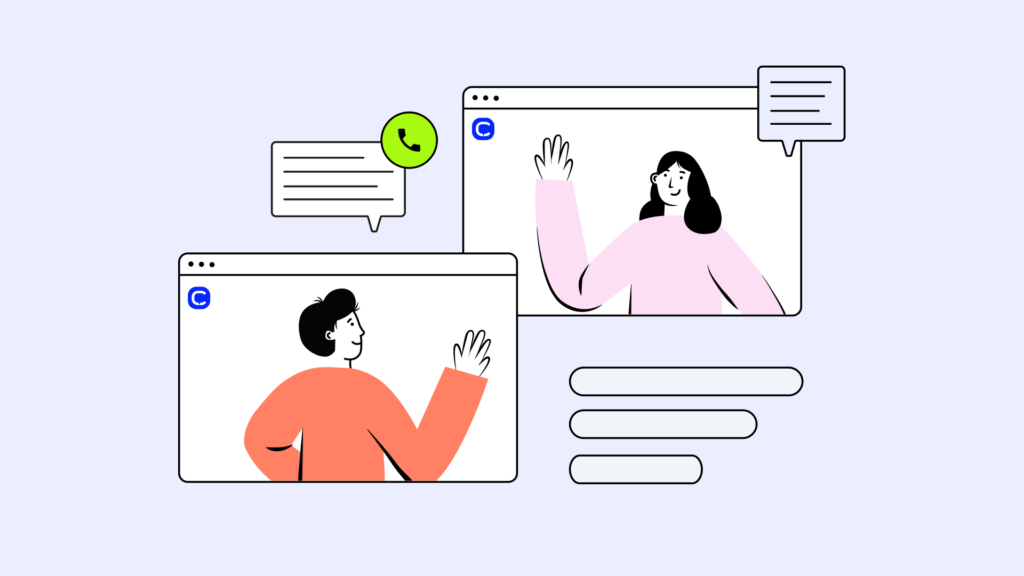
In 2025, 86% of marketers use AI-powered predictive analytics to anticipate customer behavior and optimize engagement strategies¹. This shows that artificial intelligence is no longer just a trend—it’s a tool that gives you a serious edge in retention, personalization, and revenue growth. The real question is: If your competitors are already using AI to stay ahead of their customers, why aren’t you?
Imagine knowing your customers’ next move before they make it. Sounds like science fiction? Well, thanks to AI customer behavior prediction, it’s becoming an everyday reality.
Whether you’re reducing churn, identifying upsell potential, or improving call outcomes, modern AI tools can go beyond historical analysis—they can forecast what’s next. And that’s game-changing.
In 2025, many platforms make this shift accessible. With features like Call Intelligence, Sentiment Analysis, and AI Voice Agents, they will transform your call center into a predictive engine that empowers sales, support, and marketing teams to act faster and smarter.
Key Takeaways
- Predictive analytics allows you to act before a customer churns, upgrades, or disengages—reducing guesswork across sales, support, and marketing.
- AI tools like Smart Notes, Sentiment Analysis, and AI Dialers surface emotional cues and behavior patterns in real time, so your team always knows who to engage and how.
- Predictive AI empowers personalization at scale and automates insights like call summaries and journey stages—saving time without losing the human touch.
- Implementing AI customer behavior prediction doesn’t require data science expertise. With platforms like CloudTalk, SMBs can start small and scale predictive insights over time.
- Real-world results show it works: businesses like My Mortgage Finder cut manual workload by 50% using AI-powered call summaries and insights.
Are you ready to read your customers’ minds? Then start your CloudTalk 14-day trial for free now.
The Growing Importance of Customer Predictive Analytics
Just a few years ago, using artificial intelligence to predict customer behavior felt like something reserved for tech giants with deep R&D budgets. Fast-forward to 2025, and AI’s seemingly futuristic edge has become a competitive necessity, especially for small to medium-sized businesses. AI is no longer a luxury—it’s a frontline driver of revenue growth, customer retention, and operational efficiency.
Today’s businesses have access to more customer data than ever, from call transcripts and support tickets to clickstream activity and historical purchase behavior. But raw data alone doesn’t close deals or reduce churn. That’s where AI-powered predictive analytics comes in: it connects the dots, translating customer signals into forward-looking insights you can act on.
Predictive models powered by AI help you spot when a customer is about to upgrade, go quiet, or churn—before they even say a word! And for voice-centric businesses, the shift is even more significant.
CloudTalk’s AI Voice Agents and AI Smart Notes analyze conversations in real time to give your teams a deeper layer of context. These tools listen for emotional tone, behavioral patterns, and signals of intent, whether it’s a frustrated pause or an enthusiastic upsell cue. Consequently, sales, customer success, and marketing teams can use these insights to optimize their messaging, timing, and focus—all in an automated way.
So why is this so important right now?
- Sales teams can zero in on leads with the highest conversion or upsell potential.
- Customer success teams can proactively flag issues before they escalate.
- Marketing teams can deliver hyper-personalized messages at the right moment in the buyer journey.
Mind that using AI to predict consumer behavior isn’t just for B2C. A recent study shows that B2B companies using predictive analytics of customer behavior outperform competitors by 2.9x in revenue growth.2 This is a clear signal that predictive customer service AI is quickly becoming the standard that helps businesses redefine (not just) sales and customer service.
Start growing like crazy with AI. Sign up for CloudTalk’s free 14-day trial and leave your competition behind.
What Is AI-Powered Customer Behavior Analytics?
AI-powered customer behavior analytics is the process of using artificial intelligence to study, interpret, and predict how customers are likely to act. This AI customer behavior analysis is based on historical trends, real-time behavior, and contextual signals.
Put simply, it’s where consumer artificial intelligence meets actionable business insight.
To gather those insights, AI models draw from a variety of data sources, including:
- Historical data: purchase history, support tickets, subscription activity, login frequency.
- Behavioral signals: clickstreams, scroll depth, page views, bounce rates, session duration.
- Contextual inputs: time of day, location, device type, even weather data.
- Conversational insights: tone, pace, keywords, and sentiment extracted from calls and chats.
These combined data points allow AI systems to detect patterns that are both:
- Macro-level (e.g., standard churn signals across your customer base).
- Micro-level (e.g., an individual customer showing high purchase intent).
Thanks to AI-powered call centers, you can now integrate this intelligence directly into your workflows. Adopt customer behavior monitor features like Transcript Search, Call Recording, and Talk-to-Listen Ratio, and act on behavior trends without manual guesswork.
This is the backbone of modern predictive analytics in customer service—helping sales and support teams work smarter, faster, and with better results. And this is exactly what the best AI call center software helps you achieve!
Tired of wasting time on manual call reviews and refund disputes? Check out how we helped My Mortgage Finder … and how we can help you too.
Why AI Customer Behavior Predictions Are Important for Business
Timing and personalization often determine whether a customer converts, renews, or churns. That’s where AI customer behavior prediction becomes a game-changer.
By analyzing patterns across voice, text, and digital touchpoints, AI unlocks a new level of responsiveness, personalization, and precision. This empowers businesses to act before customers make decisions, not after.
AI predictive intelligence is really powerful, especially for SMBs. It helps you personalize at scale, reduce churn, optimize revenue, boost operational efficiency, and even map out your customers’ journey (but more on that later).
In short, predictive analytics improves your processes and future-proofs your business. Pair it with smart tools like AI dialers, and you’ve got yourself foundations for a more intelligent, agile operation.
How to Predict Consumer Behavior with AI in 2025
Predicting customer behavior with AI is about creating a connected system of data, intelligence, and automation.
To do this effectively in 2025, businesses must combine first-party data, machine learning, natural language processing (NLP), and even integrated voice platforms like CloudTalk.
AI-powered solutions aren’t enough, though. If you want to build a predictive strategy that delivers results, you must incorporate the individual strategies outlined below.
Leverage First-Party Data to Build Behavioral Models
Your most valuable data already lives inside your ecosystem—it just needs structure.
Start by gathering high-quality customer insights from:
- Your CRM
- Website interactions (pages visited, time spent, forms completed)
- Customer support tools
- Call center platforms like CloudTalk, where you can mine call recordings, notes, and tags
Start feeding data you’ve got into AI models to predict outcomes like purchase probability, upsell potential, and churn risk. Start making decisions smarter.
Apply Machine Learning to Segment and Target Audiences
Using clustering algorithms or decision trees, you can automatically group customers by:
- Purchase behavior
- Engagement level
- Likelihood to convert or churn
These insights fuel predictive marketing research, allowing your team to deliver highly personalized campaigns that increase ROI and conversion rates. With a top-notch AI Cold Calling Software and proper segmentation, even outbound efforts can become highly efficient.
Integrate Real-Time Analytics for Adaptive Customer Journeys
As consumer behavior isn’t static, your strategy shouldn’t be either. CloudTalk enables real-time monitoring through features like:
Give your teams the tools they need, help them adapt to customers’ needs, and make them more successful. They will thank you.
Use NLP for Sentiment and Intent Analysis
Natural Language Processing (NLP) allows you to extract rich, actionable insights from:
- Customer conversations
- Chat transcripts
- Post-call surveys and support tickets
NLP can detect emotions like frustration or excitement and extract intent, such as interest in a product, need for help, or risk of churn. Feeding these insights back into your systems improves both your products and your support strategy.
Automate Churn Prevention with Predictive Dashboards and AI Agents
Don’t just analyze—act before it’s too late. With AI-powered suites like CloudTalk, you can set up predictive dashboards that surface key churn indicators like:
- Churn probability
- Customer Lifetime Value (CLV)
- Engagement drop-offs or inactivity trends
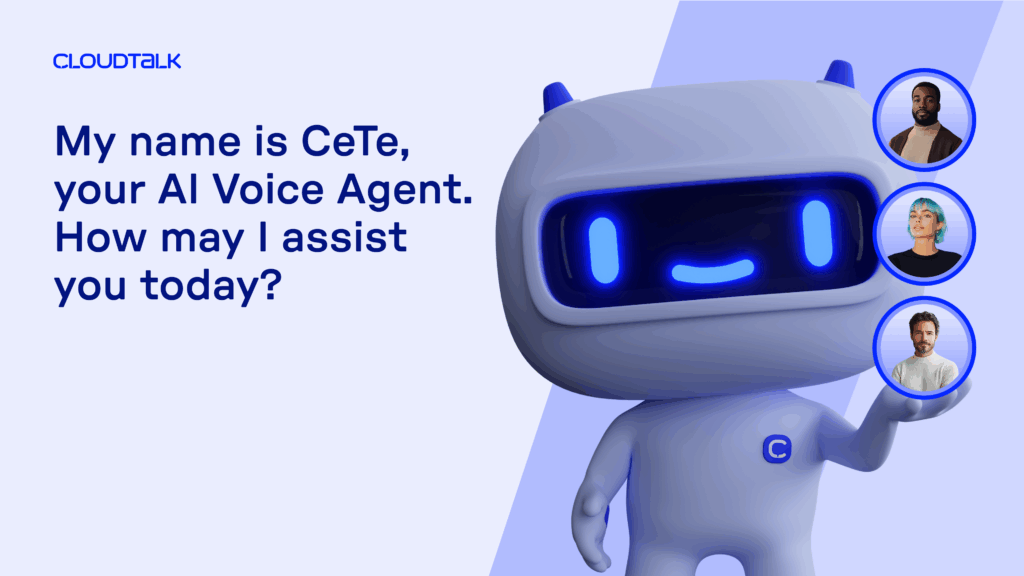
AI predicts churn by spotting subtle patterns in customer behavior, such as declining call frequency, shorter interactions, negative sentiment, or missed follow-ups, long before a customer officially disengages. These signals help you intervene early and retain more customers.
Once high-risk customers are identified, tools like Auto Answer and AI Phone Answering Service help automatically trigger timely responses—from proactive support calls to follow-up emails or priority routing to a human agent.
Curious what proactive AI assistance might cost your business? Here’s a transparent look at answering service pricing tailored to SMBs ready to scale.
What Customer Behavior Patterns Can Be Predicted with AI
AI doesn’t just analyze what your customers are doing—it tells you what they’re about to do, and why it matters.
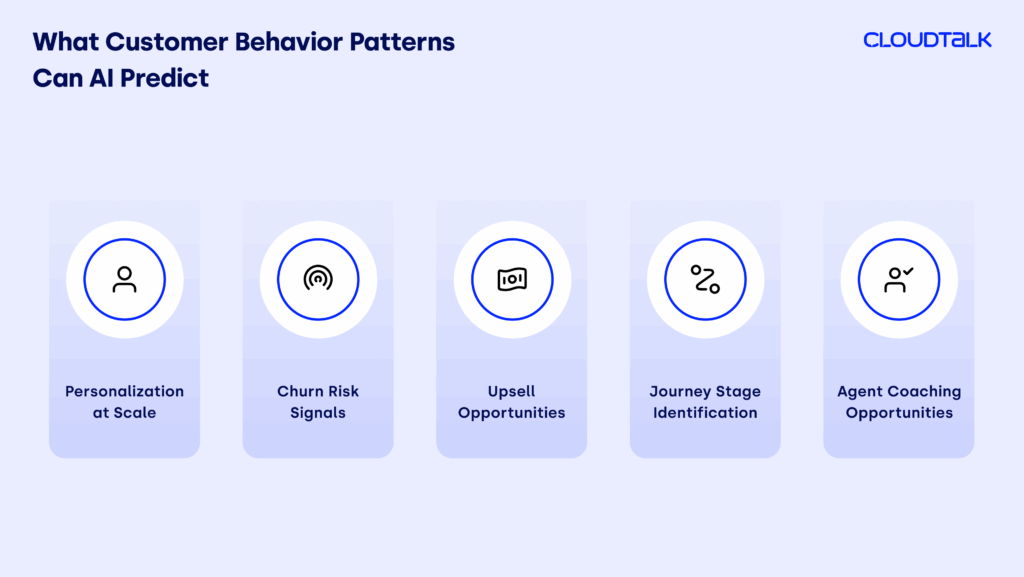
Here are five critical patterns AI can help you identify, and how CloudTalk supports each one:
- Personalization at Scale: AI enables dynamic, 1:1 personalization, from routing calls to familiar agents to customizing outreach messages based on customer history and preferences. CloudTalk’s Preferred Agent feature ensures customers speak with the same rep whenever possible, creating more relevant, emotionally resonant interactions that improve satisfaction and loyalty.
- Churn Risk Signals: Behavioral red flags like reduced call engagement, shorter responses, or repeat issues can indicate dissatisfaction. AI identifies these signals automatically, allowing small and medium-sized businesses to intervene before customers leave. Check out how even small companies can detect and prevent customer churn early.
- Upsell Opportunities: AI-powered voice agents can detect subtle signals of interest like keywords, tone shifts, or repeated questions about specific features. These insights help sales teams identify high-value leads and cross-sell or upsell at the perfect time. This is another reason why SMBs are turning to AI Voice Agents such as the ones designed by CloudTalk.
- Journey Stage Identification: Is your customer in onboarding, stuck in consideration, or ready to renew? AI maps real-time behavior across channels to pinpoint exactly where customers are in their journey at a given moment. CloudTalk’s AI Customer Service tools help teams adjust tone, messaging, and timing to match the customer’s needs, boosting conversion and retention.
- Agent Coaching Opportunities: Behavioral analytics doesn’t just apply to customers—it can enhance team performance too. CloudTalk’s Call Center Voice Analytics highlights coaching moments based on talk ratios, sentiment, and pacing.
Predict your customers’ behavior patterns like a pro. Book a demo with our AI experts to learn how.
Behind the Scenes: How Predictive Analytics Works to Detect Customer Behavior
While the impact of AI customer behavior prediction is easy to see (better conversions, lower churn, more personalized support), the engine behind it runs on multi-layered data analytics and smart automation. Let’s break down what happens behind the curtain.
The Three Layers of AI Predictive Analytics
To understand how AI predicts customer behavior, it’s helpful to break it down into three core layers, each answering a different type of question about your customers.
- Descriptive Analytics (what happened): This layer summarizes historical data to identify patterns and trends. For example, it might show that many churned customers previously had unresolved support tickets or shorter-than-average calls.
- Predictive Analytics (what will likely happen): Based on previous behaviors, AI models forecast what actions a customer might take next, whether it’s a purchase, upgrade, or churn event.
- Prescriptive Analytics (what should you do about it): Here, AI recommends or automates the next best action. In other words, it lets you know whether to escalate a support case, prioritize a lead, or assign a preferred agent.
What AI Models Are Trained On
To produce accurate predictions, AI models are trained on rich, real-world customer data, which they gather from multiple touchpoints across your operation:
- Browsing and purchasing history: Tracks product views, cart activity, purchase frequency, and time on site.
- Call transcripts from tools like Call Recording: Analyze conversation content and tone for intent, objections, or sentiment shifts.
- Campaign engagement logs: Includes opens, clicks, and responses to emails or ads, helping map buying interest.
- Customer service interactions: Measures resolution time, escalation frequency, and satisfaction levels for behavioral insights.
How the Process Works — Step by Step
Once your data is in place, here’s how the predictive analytics process typically unfolds—from collection to action.
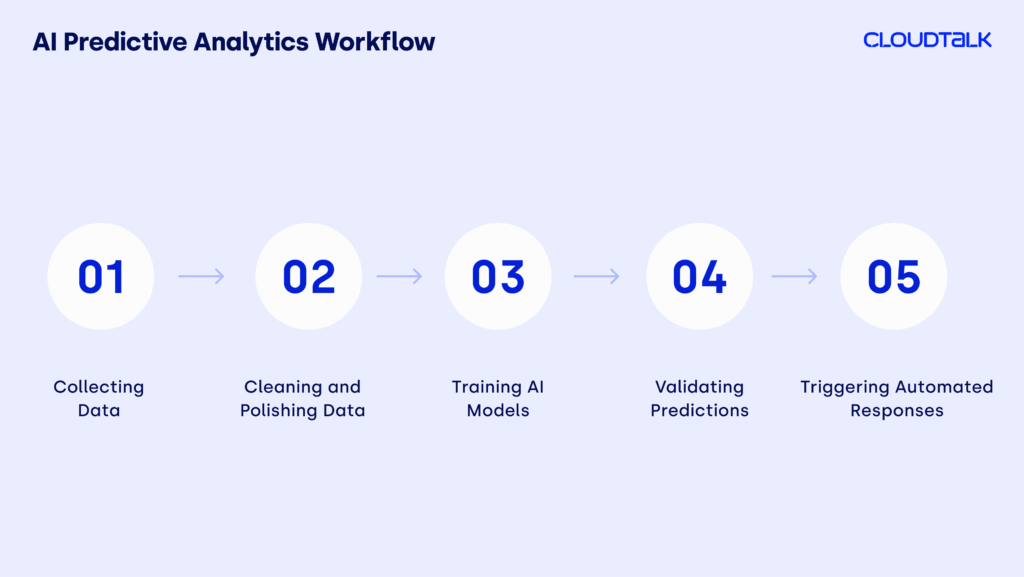
- Collect data from your CRM, website, and tools like CloudTalk.
- Clean and structure it so that it’s machine-readable and consistent across platforms.
- Train AI models using labeled examples of past customer behavior.
- Validate predictions by comparing them to real-world outcomes (e.g., Did that flagged lead convert?).
- Trigger automated responses like routing to a human agent, sending a follow-up email, or activating a proactive support workflow via AI agents.
Benefits of Predictive Analytics Customer Behavior
When businesses can anticipate what customers are likely to do next, every team becomes more proactive, personalized, and precise. Here’s how predictive analytics in customer service and sales can drive real-world impact for your company:
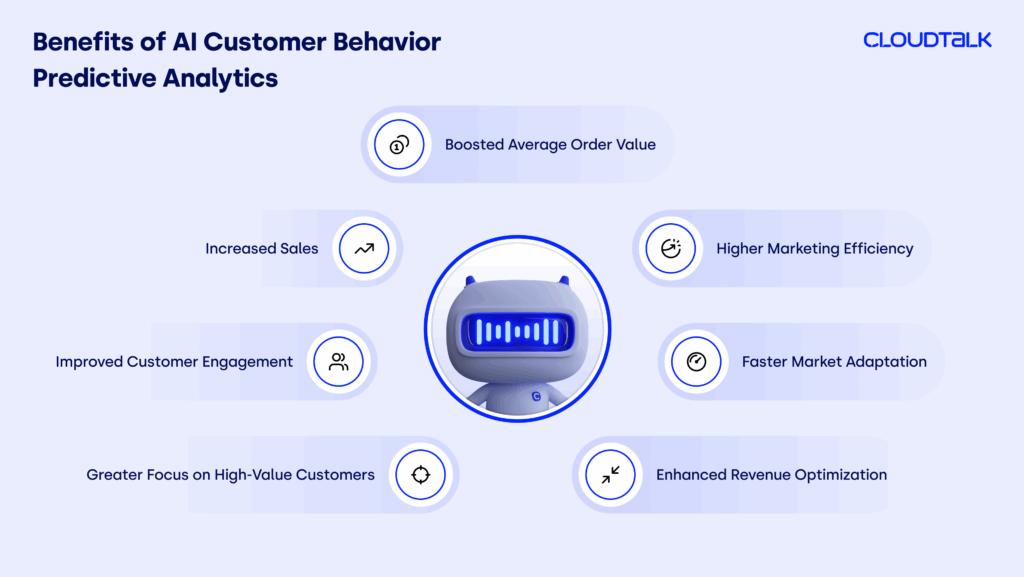
- Increased Sales: Predictive insights help your sales reps focus on leads with high purchase intent, rather than wasting time on cold or unqualified prospects. Tools like AI Smart Notes will instantly help your reps know what matters most to each lead, enabling them to close faster and smarter.
- Improved Customer Engagement: AI-powered features like Sentiment Analysis detect early signs of disengagement, like frustration in a call or reduced responsiveness. This lets your team step in before your customers step out.
- Enhanced Revenue Optimization: Not all customers are equal—some are ready to buy more, others might be at risk of churn. Nifty AI tools like Call Summary Tags help you automate lead prioritization and allocate resources to where they’ll have the biggest impact. If you want to chase revenue instead of paperwork, these tools are a no-brainer.
- Greater Focus on High-Value Customers: Predictive behavior modeling helps you spot your most profitable and loyal customers early. By identifying your VIPs, you can tailor support, offer special deals, or assign preferred agents to them. And the best thing? In the long run, this helps you build long-term loyalty and LTV.
- Faster Market Adaptation: Is a newly introduced feature being received well? Are complaints around pricing spiking? Predictive analytics sheds light on these trends before they go mainstream, allowing you to pivot faster and stay ahead of the curve. With CloudTalk, voice analytics turn your customer feedback into a real-time feedback loop.
- Higher Marketing Efficiency: AI-powered customer behavior prediction allows marketing teams to send the right message to the right audience at the right time. Unlike broad campaigns, predictive targeting ensures your budget is spent on leads most likely to convert, maximizing ROI.
- Boosted Average Order Value: When AI detects upsell or cross-sell signals, your team can jump in with relevant suggestions, increasing cart size and customer satisfaction. CloudTalk’s AI features help agents recognize and act on these opportunities mid-call or during follow-up.
Want to chase revenue instead of paperwork? CloudTalk’s free 14-day trial will get you started.
How to Implement AI Predictive Customer Analytics in Your Business
While AI seems incredibly complex (and it is), implementing AI-powered customer behavior prediction doesn’t require a data science degree. However, it requires clarity, the right tools, and a feedback-driven mindset.
Here’s a five-step framework to help your business get up and running with predictive analytics.
Define Clear Objectives
Before you dive into dashboards or datasets, get clear on what you want to predict.
- Are you trying to reduce churn?
- Do you want to spot upsell opportunities?
- Is improving post-call satisfaction scores your main target?
Setting these objectives early ensures your data strategy is focused and aligned with your business goals. For example, if your goal is churn prevention, your AI model needs access to indicators like repeat complaints, call sentiment, or resolution speed.
Choose the Right Tools
Not all AI platforms are created equal. Look for solutions that offer:
- Built-in Natural Language Processing (NLP)
- Seamless integration with your CRM, help desk, and call tools
- Real-time analytics dashboards
Here’s where CloudTalk stands out. It checks all the boxes above—and delivers so much more. CloudTalk’s features like AI Smart Notes, Sentiment Analysis, and AI Phone Answering System help SMBs start small, scale fast, and keep teams aligned.
Pro Tip: When evaluating AI tools, weigh the pros and cons of fully integrated platforms versus more isolated solutions. Explore the differences between standalone voice agents and integrated systems and make the smartest choice for your business.
Gather and Prepare Data
Your AI model is only as good as the data you feed it. Focus on collecting accurate, consistent first-party data from systems like:
- CRM (e.g., lead status, deal/pipeline velocity)
- Website (e.g., page views, form completions)
- CloudTalk (e.g., call duration, talk-to-listen ratios, transcript insights)
Oh, and don’t forget to structure and label this data to speed up model training and reduce noise.
Train and Test Models
When training and testing AI models, you no longer have to rely on data science partners, who often cost you a lot of resources. Instead, you can lean on AI-ready platforms like CloudTalk to do the heavy lifting for you … at a much lower cost!
CloudTalk’s analytics suite uses historical and real-time call data to generate smart customer behavior predictions and flag customer behavior patterns without requiring in-house model development.
Pro Tip: Start small (e.g., churn prediction), test the accuracy against real outcomes, and iterate from there.
Iterate and Improve
AI is not a “set it and forget it” solution. As customer behavior evolves, so must your prediction models.
Keep feeding new data into your system—especially from feedback loops like support surveys, call transcripts, or sales outcomes—to refine prediction accuracy over time.
Hop on CloudTalk’s 14-day trial for free. Start predicting your customers before you lose them.
Challenges to Consider When Implementing AI Predictive Analytics and How to Overcome Them
While the benefits of AI customer behavior prediction are compelling, implementation can be challenging, especially for small to mid-sized businesses. The good news? You can easily solve most of these hurdles with the right tools and approach.
Here are the four most common challenges, and how you can address them head-on.
Data Silos
Challenge: Your customer data might live in multiple systems (i.e., data silos), such as CRM, helpdesk, voice platform, and more, making it hard to get a unified view. Without integrated data, predictive models lack the context required to make accurate forecasts.
Solution: Break down the silos. CloudTalk offers integrations that seamlessly connect with CRMs and support platforms, ensuring that voice, behavioral, and transactional data flow into one analytics pipeline. Centralizing your systems makes it easier to train models and trigger meaningful actions across the customer journey.
Model Bias and Inaccuracy
Challenge: If your training data is outdated, unbalanced, or lacks diversity, your AI predictions could lead to poor targeting, missed signals, or even reputational risk.
Solution: Make data auditing a routine process. Review the quality of your training sets regularly and compare predictions against real-world outcomes. CloudTalk’s analytics give you transparent access to call-level insights, allowing you to refine models continuously and ensure ethical, high-performing AI.
Limited Internal Expertise
Challenge: Many SMBs don’t have data scientists on staff, which can make implementing predictive models intimidating or out of reach.
Solution: Start simple. Tools like CloudTalk offer real-time dashboards and built-in analytics that automatically provide predictive insights. This allows your team to act on AI-driven signals from day one, without a steep learning curve and a need for technical know-how.
Cost and Scalability Concerns
Challenge: AI solutions can sound expensive, and there’s often uncertainty about whether the investment will pay off.
Solution: Pilot your predictive analytics with a specific use case (e.g., churn reduction or lead prioritization). CloudTalk’s flexible pricing and modular features let you start small, measure results, and scale when ready. This approach lowers risk while helping you prove ROI before going all-in.
Adopting AI doesn’t mean you have to overhaul everything at once. With proper strategic planning and the right tools, even small businesses can unlock enterprise-level insights and results.
Don’t Just React to Your Customers, Predict and Win Them with AI
The companies winning in 2025 aren’t the ones reacting fastest—they’re the ones predicting first.
The good news? You can still join the winning side!
You just have to find the right tool, one that helps you know your customers and spot their needs and frustrations before they say a word. With CloudTalk, that level of foresight is finally accessible to everyone, including SMBs.
Here’s the hard truth: data is only valuable if it turns into action. CloudTalk doesn’t just give you the data—it gives you the AI-powered prediction tools to act on it in real time. It’s an all-you-will-ever-need suite to help you stay ahead, not just of your customers, but your competitors too.
Take advantage of AI and join the winning side today. Learn how CloudTalk can help you accomplish that.
Sources:
FAQs about AI customer behavior prediction
Can AI predict customer behavior?
Yes, AI can predict customer behavior. AI models can analyze large volumes of behavioral data (e.g., purchase history, tone of voice), and forecast what a customer is likely to do next.
How can AI predict customer churn?
AI can predict customer churn by identifying key behavioral signals like declining call engagement, longer resolution times, or negative sentiment in calls.
Is there an AI that can make predictions?
Yes, there is AI that can make predictions. The best AI Customer Service Solutions help you predict your customers’ next steps based on their sentiment level, response times, or a shift in the way they talk.
Can consumer behavior be predicted?
Yes, consumer behavior can be predicted. Use AI-powered call centers such as CloudTalk and build a comprehensive profile of each customer’s likely next step.
What is a predictive model of customer behavior?
A predictive model of customer behavior is an AI system trained on your customers’ past actions to forecast future outcomes.